What Is a Factorial Design? Definition and Examples
A factorial design is a type of experiment that involves manipulating two or more variables. While simple psychology experiments look at how one independent variable affects one dependent variable, researchers often want to know more about the effects of multiple independent variables. How a Factorial Design Works Let’s take a closer look at how a…
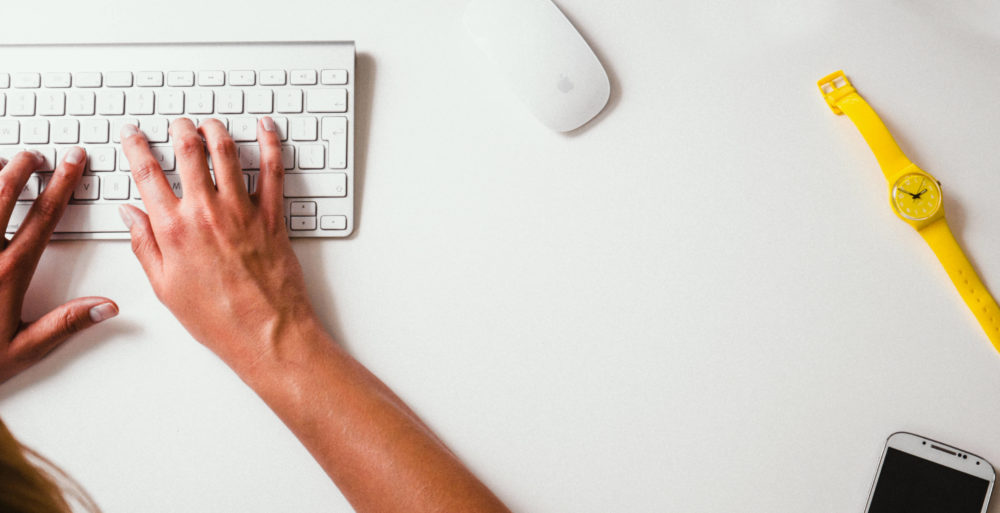
A factorial design is a type of experiment that involves manipulating two or more variables. While simple psychology experiments look at how one independent variable affects one dependent variable, researchers often want to know more about the effects of multiple independent variables.
How a Factorial Design Works
Let’s take a closer look at how a factorial design might work in a psychology experiment:
- The independent variable is the variable of interest that the experimenter will manipulate.
- The dependent variable is the variable that the researcher then measures.
By doing this, psychologists can see if changing the independent variable results in some type of change in the dependent variable.
For example, imagine that a researcher wants to do an experiment looking at whether sleep deprivation hurts reaction times during a driving test. If she were only to perform the experiment using these variables–the sleep deprivation being the independent variable and the performance on the driving test being the dependent variable–it would be an example of a simple experiment.
However, let’s imagine that she is also interested in learning if sleep deprivation impacts the driving abilities of men and women differently. She has just added a second independent variable of interest (sex of the driver) into her study, which now makes it a factorial design.
Types of Factorial Designs
One common type of experiment is known as a 2×2 factorial design. In this type of study, there are two factors (or independent variables), each with two levels.
The number of digits tells you how many independent variables (IVs) there are in an experiment, while the value of each number tells you how many levels there are for each independent variable.
So, for example, a 4×3 factorial design would involve two independent variables with four levels for one IV and three levels for the other IV.
Advantages of a Factorial Design
One of the big advantages of factorial designs is that they allow researchers to look for interactions between independent variables.
An interaction is a result in which the effects of one experimental manipulation depends upon the experimental manipulation of another independent variable.
Example of a Factorial Design
For example, imagine that researchers want to test the effects of a memory-enhancing drug. Participants are given one of three different drug doses, and then asked to either complete a simple or complex memory task.
The researchers note that the effects of the memory drug are more pronounced with the simple memory tasks, but not as apparent when it comes to the complex tasks. In this 3×2 factorial design, there is an interaction effect between the drug dosage and the complexity of the memory task.
Understanding Variable Effects in Factorial Designs
So if researchers are manipulating two or more independent variables, how exactly do they know which effects are linked to which variables?
“It is true that when two manipulations are operating simultaneously, it is impossible to disentangle their effects completely,” explain authors Breckler, Olson, and Wiggins in their book Social Psychology Alive.
“Nevertheless, the researchers can explore the effects of each independent variable separately by averaging across all levels of the other independent variable. This procedure is called looking at the main effect.”
Examples of Factorial Designs
A university wants to assess the starting salaries of their MBA graduates. The study looks at graduates working in four different employment areas: accounting, management, finance, and marketing.
In addition to looking at the employment sector, the researchers also look at gender. In this example, the employment sector and gender of the graduates are the independent variables, and the starting salaries are the dependent variables. This would be considered a 4×2 factorial design.
Researchers want to determine how the amount of sleep a person gets the night before an exam impacts performance on a math test the next day. But the experimenters also know that many people like to have a cup of coffee (or two) in the morning to help them get going.
So, the researchers decided to look at how the amount of sleep and caffeine influence test performance.
The researchers then decided to look at three levels of sleep (4 hours, 6 hours, and 8 hours) and only two levels of caffeine consumption (2 cups versus no coffee). In this case, the study is a 3×2 factorial design.
Sources:
Baker TB, Smith SS, Bolt DM, et al. Implementing clinical research using factorial designs: A primer. Behav Ther. 2017;48(4):567-580. doi:10.1016/j.beth.2016.12.005
Collins LM, Dziak JJ, Li R. Design of experiments with multiple independent variables: a resource management perspective on complete and reduced factorial designs. Psychol Methods. 2009;14(3):202-224. doi:10.1037/a0015826
Haerling Adamson K, Prion S. Two-by-two factorial design. Clin Simul Nurs. 2020;49:90-91. doi:10.1016/j.ecns.2020.06.004
Watkins ER, Newbold A. Factorial designs help to understand how psychological therapy works. Front Psychiatry. 2020;11:429. doi:10.3389/fpsyt.2020.00429